Key Components for Building a Successful Demand Forecasting Solution
Tue Oct 08 2024

Author
Sumith Matharage
Creating an effective demand forecasting solution requires much more than selecting the right algorithm. Success lies in a combination of robust workflows and thoughtfully designed architecture. These core components work together to ensure the solution delivers meaningful, actionable insights that drive business value. In the following sections, we outline the essential elements of a successful demand forecasting system, focusing on the critical aspects of both workflow and architecture.
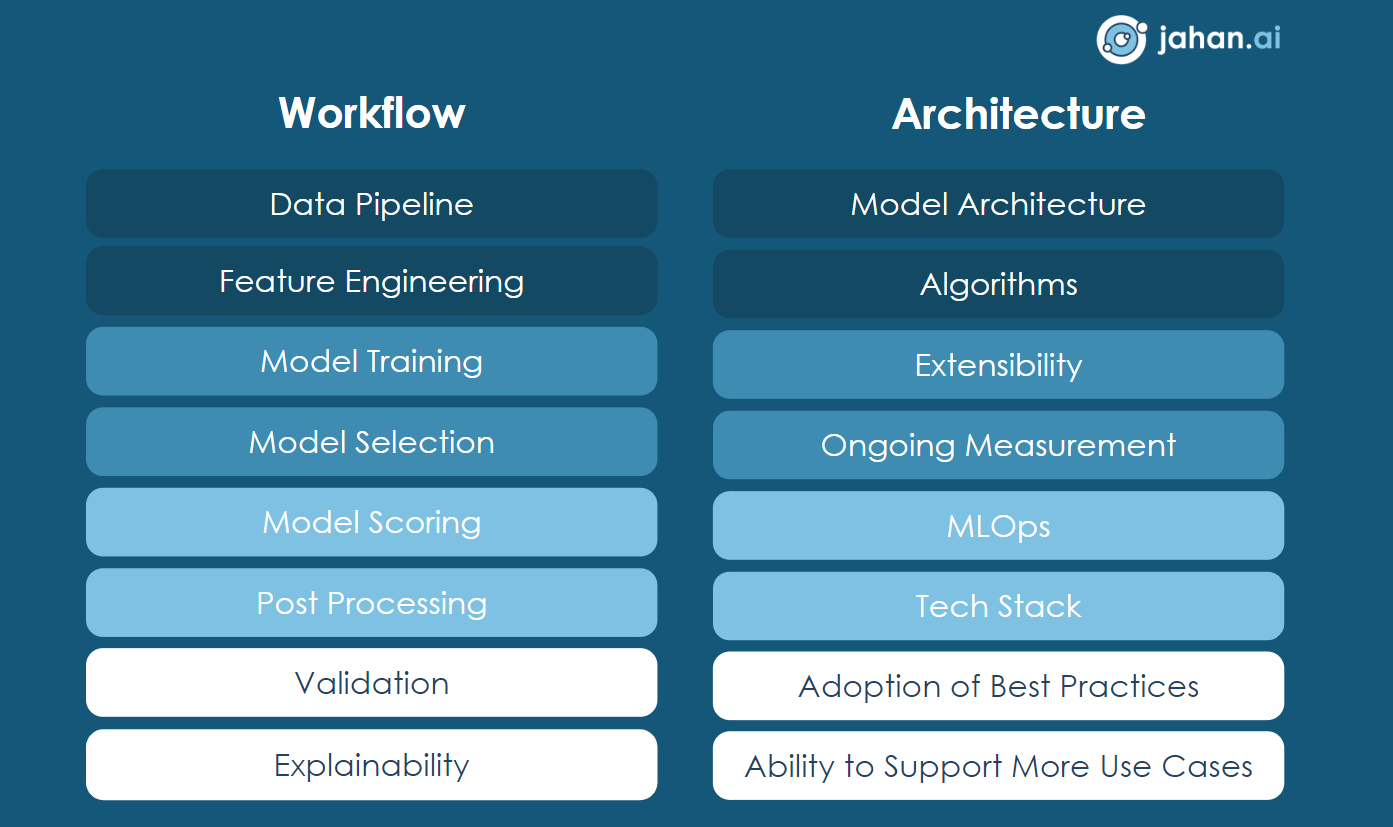
Core Components of a Successful Demand Forecast
Workflow Components:
The workflow of a demand forecasting solution involves a series of interconnected processes that transform raw data into actionable forecasts. Each step is crucial for ensuring that the predictions are accurate, relevant, and useful. Key elements of the workflow include:
Data Pipeline: The data pipeline should integrate both historical and forward-looking data feeds, ensuring that data is properly cleansed and validated. Implement robust monitoring and alerting systems to quickly identify and address data quality issues, ensuring the integrity and consistency of the input data for accurate forecasting.
Feature Engineering: Feature engineering involves creating a framework that extracts valuable insights from raw data, transforming it into meaningful variables that can better represent the underlying patterns. This process is crucial for uncovering complex relationships within the data that may not be immediately apparent. By leveraging advanced feature engineering techniques, businesses can significantly enhance the predictive power of their models and improve forecast accuracy.
Model Training: Model training involves learning the relationships between input variables (features) and the target variable (demand). The model must be fine-tuned to optimise performance, balancing both accuracy and computational efficiency. The right level of tuning can reduce operational costs by more than half without compromising model accuracy.
Model Selection: Model selection involves choosing the most appropriate model based on its performance on a holdout set - a portion of the data not used during training but reserved for testing. This process ensures that the selected model generalises well to unseen data and maintains robust predictive capabilities. By evaluating how well different models perform on this holdout set, businesses can select the model that is best suited for accurately forecasting future demand while avoiding overfitting to the training data.
Model Scoring: Model Scoring enables you to generate predictions using a trained model. The right combination of batch and realtime forecasting interfaces is essential to meet both operational use cases and scenario-planning use cases.
Post-Processing: Post-processing refers to the final step of refining model predictions to ensure they are practical and actionable. After generating raw predictions from the model, post-processing ensures that these predictions fall within a reasonable range, such as between 0 and a defined upper limit. This helps to keep the forecast in check, avoiding any unexpected operational impacts.
Validation: Validation is a critical step in the demand forecasting process that involves thoroughly assessing a model’s performance. Comprehensive validation helps businesses gain key insights into how well the model is functioning. By conducting a thorough validation, businesses can identify the strengths and weaknesses of the forecast, enabling targeted improvements and fine-tuning of the model.
Explainability: Explainability refers to the ability to understand and interpret the factors influencing a model's predictions. It offers insights into the 'why' behind the forecast, enabling businesses to identify key drivers, review the forecast outlook, and deep dive into model performance. This transparency ensures that businesses can trust and act on the predictions with greater confidence.
Architecture Components:
The architecture of a demand forecasting solution provides the technical foundation that supports the entire process. A well-designed architecture ensures scalability, flexibility, and long-term sustainability of the system. The key elements of architecture include:
Model Architecture: A robust model architecture leverages the strengths of advanced feature engineering combined with the ensembling of multiple algorithms to provide extensive coverage of the forecasting solution. This approach ensures that the model is comprehensive, adaptable, and capable of delivering precise and reliable forecasts.
Algorithms: A well-designed forecasting solution utilises a thoughtful combination of both traditional and modern algorithms. By integrating these approaches, the forecasting model leverages the strengths of each. Traditional algorithms offer robustness and simplicity for well-understood patterns, while modern machine learning techniques provide flexibility and advanced capabilities for handling complex or dynamic data scenarios. This synergy results in a more comprehensive and accurate forecasting solution.
Extensibility: Extensibility refers to the capacity of a forecasting model to be expanded or enhanced over time to adapt to new data, changing requirements, or evolving business needs. By incorporating extensibility, a forecasting model can evolve with the business environment, maintaining its effectiveness and value over the long term.
Ongoing Measurement: Ongoing Measurement involves the continuous evaluation and tracking of the value delivered by a forecasting model. It extends beyond simply assessing the model's performance for accounting purposes, focusing on value realisation, process improvement, and learning and adaptation. Implementing ongoing measurement allows businesses to keep their forecasting efforts aligned with their goals and optimised for maximum value and accuracy.
MLOps: MLOps encompasses the practices and tools used to manage and maintain machine learning models in production environments. This includes ongoing production support, model maintenance, operational efficiency, and collaboration and governance. MLOps ensures that forecasting models continue to deliver value and remain effective throughout their lifecycle, supporting sustained accuracy and reliability in production settings.
Tech Stack: Tech Stack encompasses the fundamental set of technologies, including the core platform and tools, required to execute and manage the forecasting process. These components collectively provide the necessary environment and resources to effectively implement and maintain forecasting solutions, ensuring they are executed efficiently and accurately.
Adoption of Best Practices: Leveraging modern machine learning and engineering practices and design patterns is crucial. By adopting these best practices, businesses can ensure that their forecasting models are developed using the most effective, efficient, and up-to-date methods, leading to improved performance and adaptability.
Ability to Support More Use Cases: A forecasting model’s ability to address a broad range of applications across the entire value chain is essential. By accommodating various use cases, the model can deliver valuable insights throughout the value chain, thereby enhancing overall business performance and decision-making.
It is essential for businesses to focus on these key components in both workflow and architecture to develop a demand forecasting solution that is accurate, reliable, scalable, and adaptable to future demands. Our Self-Assessment Scorecard can help you evaluate the current state of your demand forecasting efforts and guide your next steps. Below is a recent assessment for one of our clients, which evaluated the effectiveness of their current solution. The evaluation revealed significant gaps across most key components, providing insights for planning the next steps in their forecasting journey.
Self-Assessment Scorecard:
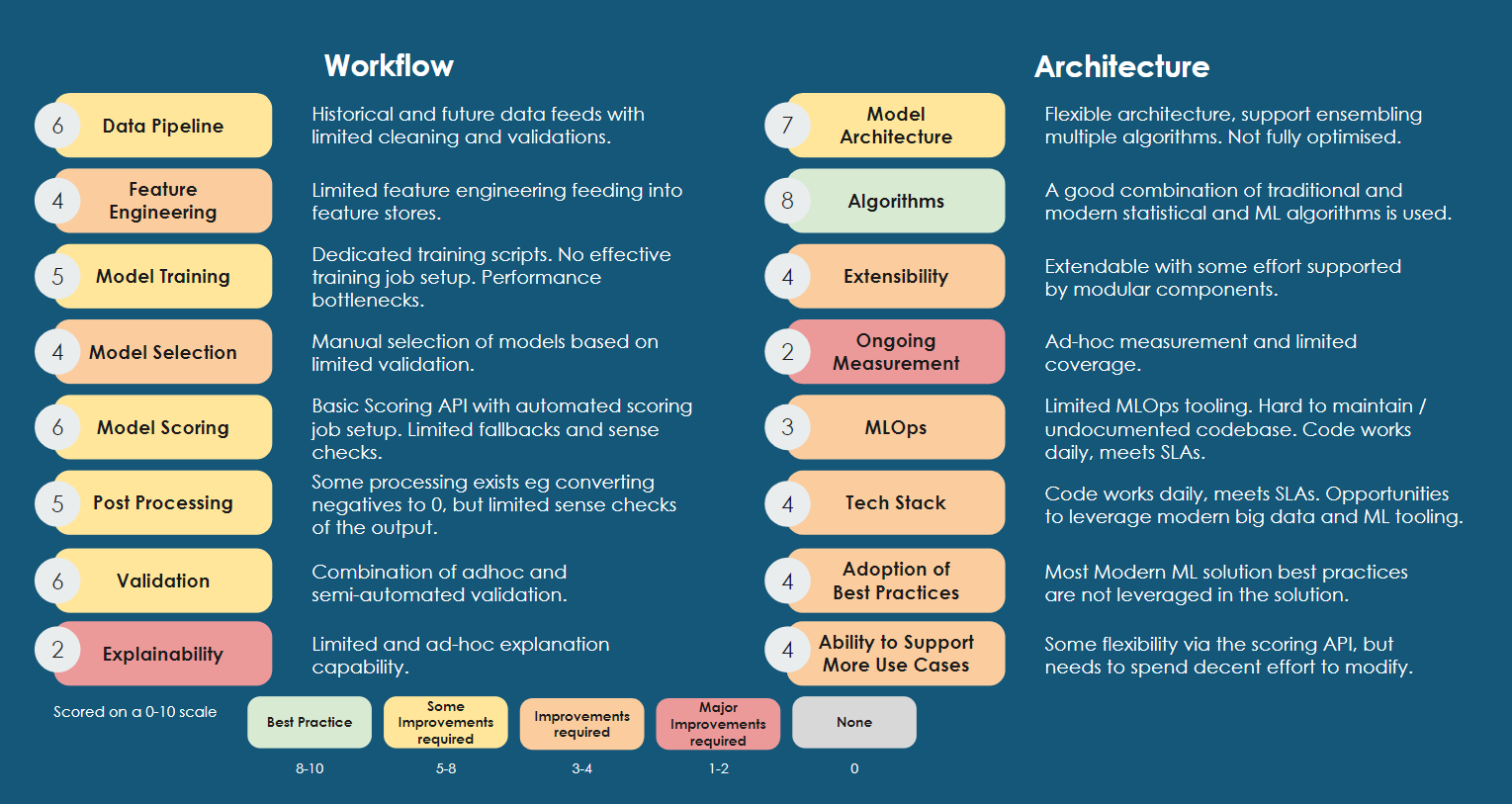
Example assessment scorecard
#demandforecasting #machinelearning #ai #architecture #jahanai #forecastworkflow #selfassessment
At jahan.ai, we build end-to-end AI twins for retail and supply chain businesses. We take the concept of a digital twin, a virtual replica of business processes, further with advanced AI, which not only mirrors these processes but also automatically optimises them. In this way, we support businesses in driving efficiency, going beyond their potential.