Author
Alok Joshi
An AI Twin, much like a digital twin, is a virtual model of a physical system or process, that leverages AI to not just mirror it but also predict, learn, and optimise it. Walmart, for example, uses digital twin technology to simulate store layouts, and use AI to simulate product placement and aisle arrangements to find the optimal customer friendly setup. In the world of Retail and Supply Chains, there are a lot of applications and benefits to unpack with this capability.
From Digital Twin to AI Twin
The concept of an AI twin stems from an evolution of a “digital twin”, which has a strong history and application in science and engineering. In order to truly appreciate the power of an AI Twin, it's worth understanding the origin and success of digital twins.
Digital Twin
Digital twins date back to the 1960s, when NASA used a replica spacecraft to save the astronauts on board the Apollo 13 from an exploded oxygen tank. The use of test and learn scenarios until they solved the problem of how to let the crew continue breathing was the beginning of using simulations in a virtual environment, a digital twin.
Since then we’ve seen broad applications across all industries:
- To minimise mistakes and costs: F1 racing teams simulate changes to their car before actually modifying the vehicle
- To detect failures: Amazon use IoT to create a replica of their supply chain, real-time, to predict machine failure and take proactive measures
- Save on massive capital costs: Airlines simulate airflow instead of needing expensive experiments in air tunnels
- Save lives: vital organs have been replicated, and treatments simulated, predicting the most likely successful course of action
In short, digital twins make it quicker, cheaper and more accurate to make decisions that can have significant benefit or avoid disaster.
The Evolution to AI Twin
One thing that is common with all digital twins is the data needed to create these simulations - and lots of it. With the rapid development of AI and Machine Learning capabilities, it has become more feasible to now analyse this large volume of data, learn from it and automate actions to take (not just gain insights).
Enter the AI Twin. Simulation is the first step, but how do you know what the optimal levers and settings are to get the best results? You can test and learn manually with millions of combinations, or you can use AI to automatically optimise each one for you.
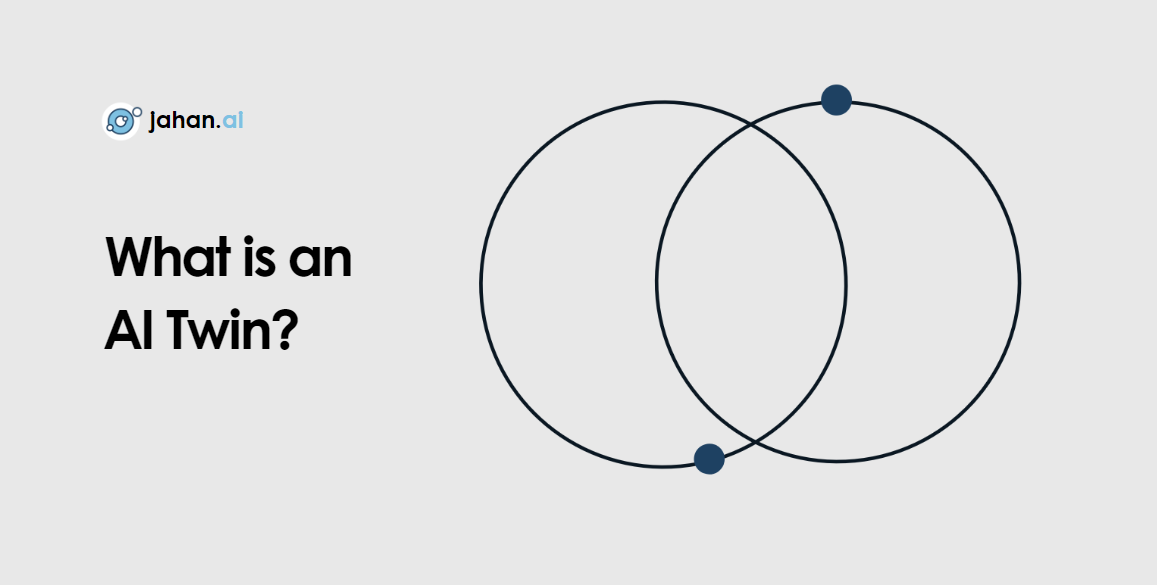
jahan.ai AI Twin
So what are the applications in Retail and Supply Chains?
Whether your objectives are to maximise sales, reduce waste, optimise margin, increase customer satisfaction or a balance of all, an AI Twin can simulate the right business process changes needed to meet your goal. Imagine these as objectives that you could simply slide to the desired setting, then visualise what changes your business needs to take in order to meet them.
Let’s look at some examples to bring it to life:
In Supply Chain
Problem: What is the optimal amount of inventory to hold in each warehouse to reduce costs while maintaining great availability?
Considerations when changing inventory:
- Forecasted demand for each product (jahanForecast)
- Customer satisfaction (jahanCustomerAnalytics)
- inventory capacity and predicted availability (jahanInventory)
- Vehicle capacity to move the goods (jahanTransport)
- Order plan changes (jahanOrderPlan)
- Labour to maintain stock levels (jahanLabour)
How can an AI Twin help? Across each of these considerations, we’ve listed the associated jahan AI model to help predict and optimise the answer. What if these models are interconnected in an “AI Twin” of your business? You could simulate and optimise every decision along the way e.g. change a lever in labour if its getting too expensive, see the impact on inventory in a specific location. Optimise before you execute.
In Commercial Retail decisions
Problem: New product range for summer. What is the optimal mix to maintain margins?.
Considerations when planning assortments:
- Forecasted demand of new products (jahanForecast)
- Customer response (jahanCustomerAnalytics)
- Placement and cannibalisation of existing range (jahanRange)
- Pricing to meet margin target (jahanPricing)
- Promotions to launch and who to target (jahanPromo + jahanPersonalisation)
- Order plan changes (jahanOrderPlan)
- Transportation costs (jahanTransport)
- Changes to rosters (jahanLabour)
How can an AI Twin help? As you can see, simulating adding and removing products in your assortment and assessing the end-to-end impact would be very powerful. It could make the difference between a successful assortment that exceeds your profit targets, to having adverse customer impacts that damage brand and reputation.
So while digital twins may have been around for some time, recent developments in AI have allowed us to effectively turbo-charge the virtual environment, providing the ability to find optimal settings and fine-tune them automatically.
At jahan.ai, we think of problems strategically and holistically, which is why we develop AI Twins, allowing you to see end-to-end impacts of every decision in the retail and supply chain value chain. From commercial planning to direct customer impacts, our AI models create a “control tower” to globally optimise based on the objectives and problems we need to solve. The applications in end-to-end retail are endless, and although we’ve provided a couple of examples - I’m sure you would be able to think of countless more. What would you like to simulate?
aiTwin
demandForecasting
retail
supplyChainPlanning